How to Apply Statistical Techniques in a Quality Management System (QMS)
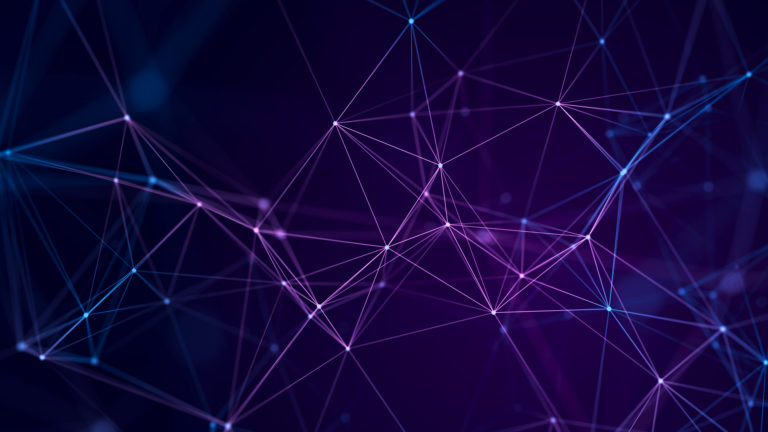
21 CFR 820, Subpart O, the last subpart of the Quality System Regulation, almost appears to be an afterthought. The first clause states that statistics should be used as appropriate, and the second clause discusses sampling plans, if used. It makes one think that statistics aren’t really important for compliance to the regulation, and, indeed, this clause is seldom cited directly in 483 observations.
However, the FDA does expect the use of quality techniques, including the use of statistics to guide risk-based, science-based decision-making. There is no better way to demonstrate that decisions were made using objective criteria than to establish a statistical method to evaluate the problem.
The good news is that statistics need not be difficult. It is the objectivity that matters in the statistical techniques. So, for example, if performing a trend analysis, if your trend chart plots data in a way to graphically show that the data are either stable or trending upward or downward. This may be sufficient, without necessarily applying all SPC runs / rules or ensuring a larger-than-available data set before commencing trend analysis.
Run charts are probably the most useful statistical tool in a quality management system because run charts are the best way to present the state of quality to Management, and in the FDA’s QSIT model download (fda.gov) Management is at the hub of all quality subsystems. Use the table below to identify run charts that might be presented at management review, and the associated quality subsystem:
Quality Subsystem | Run Chart Information |
CAPA | (Stacked bar chart per month: Nonconformances by cause: Material Controls, Production & Process Controls, Document Controls, Facility & Equipment Controls Percent NCs escalated to CAPA per month compared to number of NCs for the month # of CAPAs opened per month # of CAPAs closed per month Average CAPA age per month % of new CAPAs for a recurring issue % of CAPAs failing the Effectiveness Check |
Material Control/ Production & Process Controls | OOS per month OOT per month Training Compliance per month Supplier performance trending (on-time delivery) Supplier performance trending (purchased material conformity) |
Facility & Equipment Controls | % calibrations performed on time per month % calibrations failed on recalibration date Equipment/Systems removed from service Equipment/Systems newly qualified/implemented Systems completing validation vs. monthly goal |
From the table, you may have noticed that not every quality subsystem has its own run chart. This is because the CAPA system is so overreaching into all the other quality subsystems, and also because Design Controls, by their nature as covering products under development (not in production) have a separate session (i.e. Design Review) to track the product development process.
Because products under development still have many unknown attributes, statistical analysis is especially important in product development. In a hypothetical product development example, where two potential designs are compared for relative safety and effectiveness, a statistical model would best be able to discern which design performs better. Design reviews, for business reasons, also track the effectiveness of the product development process by evaluating for on-time completion of development milestones. Most important, product development relies heavily on data from clinical evaluations. The study design of a clinical evaluation is of utmost importance, and this is the one area where the services of a professional Statistician ensures that proof of product performance and safety are established.
Complaint handling relies heavily on trending to gauge effectiveness of the quality system. First, the number of incoming complaints per month is a good indicator of customer satisfaction, but the number of hazardous and reportable complaints per month is a good indicator of product safety. If a complaint is FDA is reportable, then it is also important to ensure that reports are sent to the FDA on time, so on-time delivery of reports, is an important metric to track, as well as the status (age, % completion) of advisory notices and recalls.
Other areas where statistics are helpful for decision-making include the evaluation of equipment useful life. While reliability engineers may calculate a Mean Time Between Failures, most medical device companies do not want to test equipment to failure to learn of its useful life. However, by plotting calibration performance and wear and tear on critical parts, the useful life of a piece of equipment can become more predictable, and servicing can be scheduled before a breakdown, ensuring production free of equipment-related nonconformities.
The FDA specifically calls out sampling plans for statistics. Most people associate sampling plans with inspection of parts or incoming materials, applying the ANSI standards Z1.4 for discrete attributes, or ANSI Z1.9 for variable data. These standards are good when associated with random sampling of product. Sampling plans can also be applied to other areas of the quality system, such as for validations, where an enhanced sampling plan would be used to ensure that the process meets the performance attributes, even if random sampling after processing during normal production verifies conformity to requirements. As a reminder, the FDA’s stance is that a process whose output cannot be fully verified by subsequent test, validation is required. Samples can never fully verify, and certainly if destructive testing is employed, validation is definitely required, (and so are statistical techniques) because testing in full will destroy the entire batch.
Sampling plans for quality testing extend beyond selection of which units to sample. Test criteria for pass/fail acceptance should also be based on statistical rationale. Gage R&R, test method validation, and specification studies are important to understand a test system’s error, and the potential range of results that may be acceptable, and the threshold at which conforming material becomes nonconforming. If testing multiple replicates in a test method, statistics need to be used to determine whether the appropriate number of replicates tested, and other statistical methods should review the scatter around replicates to determine whether the test system is sufficiently reliable to accept the result. This information is crucial to facilitate robust investigations into OOS (out-of-specification) and OOT (out-of-trend) test results. Such information is subject to quality audit because no organization wants to be accused of releasing unfit material because of a subjective decision to disregard failing test results.
Such accusations are common during FDA inspections, and quality audits are the tool to detect such nonconformities. So it comes as no surprise that quality audits use statistics too. Unlike other quality subsystems, the auditing program should apply statistics in a combination with logic, since auditing is an exercise to determine whether the auditee meets stated requirements (the letter of the regulation), and more subjectively, whether the auditee meets implied requirements (the spirit of the regulation). The decision of which quality subsystems to audit is therefore generally decided through non-statistical rationale. Statistics, however, are helpful when deciding how many records to sample for review of audit evidence. The FDA follows the same methodology.
As any auditor knows, though, records for audit should not be completely sampled at random. For example, if sampling a group of compliant records or CAPA records, the auditor will tend to sample the records that appear to have the greatest quality impact. This is because a process gap shown in high-impact records is more likely to reveal systematic quality deficiencies. Records sampled at random may not be very impactful, and the test of a good quality system is to see the response when things really start going out-of-control.
Statistics, when applied appropriately, ensure that little problems won’t snowball into major issues that may result in a host of costly product safety and customer satisfaction issues. So while 21 CFR 820, Subpart O (820.250) may be one of the shortest sections of the Quality System Regulation, risk-based, science-based thinking touches every element of a quality system.
The best way to demonstrate risk-based, science-based thinking is through application of statistics and other quantitative techniques to demonstrate the rationality behind quality-impacting decisions. Statistical tools help protect the manufacturer through application of sound decision-making, which results in impeccable quality records to present during the FDA inspection..